Harnessing the Power of AI To Optimize Drug Therapy
As artificial intelligence gains momentum in healthcare, experts see opportunities for pharmacists to harness the technology to avert drug-related mishaps and select the most optimal treatments for patients.
“We want to minimize the harm that we’re doing with medications,” Adrijana Kekic, PharmD, told attendees at the APhA2023 Annual Meeting & Exposition, in Phoenix. “We need to understand better how [to identify patients] who are going to be at high risk of unfavorable drug response events.”
Adverse drug reactions (ADRs) are estimated to be between the fourth and sixth leading causes of death worldwide (Ther Innov Regul Sci 2023;57[1]:100-103; JAMA 1998;279[15]:1216-1217; Lancet 1998;351[9110]:1183). The good news? “There is a lot being done in this space,” said Dr. Kekic, a pharmacogenomics clinical specialist and an associate program director of education for outpatient pharmacy at Mayo Clinic in Arizona. A growing body of research indicates that AI can be applied to a variety of disease states to predict which patients might benefit from a given intervention or are at heightened risk of an ADR or other negative outcome. These range from depression to opioid use disorder (OUD), kidney damage, sepsis and more (see boxes). However, there are formidable challenges to overcome before pharmacists can fully take advantage of this technology in the clinic.
ADVERTISEMENT
AI Could Tackle Big Data
“AI and machine learning gets so much attention because we are in the era of Big Data that includes large volumes and large variety,” said Wei-Hsuan “Jenny” Lo-Ciganic, MSPharm, MS, PhD, a pharmacoepidemiologist who was an associate professor in the Department of Pharmaceutical Outcomes and Policy at the University of Florida College of Pharmacy, in Gainesville, at the time of the APhA meeting (she has since moved to the University of Pittsburgh School of Medicine). “AI and machine learning could be a solution to handle Big Data.”

AI uses automatic processes to mimic human abilities, Dr. Lo-Ciganic said. One form of AI with the potential to make predictions that support clinical decision making is machine learning (ML). “You basically feed it a lot of data, and then based on the data that you fed it, it learns,” Dr. Kekic said. A subset of this technology, deep learning, also uses data to train algorithms to complete tasks. However, its algorithms simulate the functioning of the human brain to solve more complex problems for applications such as digital assistants and self-driving cars.
ADVERTISEMENT
Dr. Lo-Ciganic and her colleagues are studying ML for a variety of applications, including how medication adherence is associated with hospitalization risk in patients with type 2 diabetes (Med Care 2015;53[8]:720-728), predicting direct-acting antiviral treatment failure in patients with chronic hepatitis C (Hepatology 2022;76[2]:483-491), and predicting OUD and overdose risk.
Mayo Clinic researchers have investigated using ML to predict rheumatoid arthritis disease activity based on plasma metabolite abundance levels and treatment response to methotrexate (Arthritis Res Ther 2021;23[1]:164; Arthritis Care Res[Hoboken] 2022;74[6]:879-888). By using this approach, Dr. Kekic said, “we are kind of minimizing that trial-and-error prescribing … and hopefully treating the patient more precisely.”
One promising area for AI integration is pharmacogenomics. “Machine learning and deep learning are important because we can stratify patients according to their risk factors in disease intensity, and then we can predict these unfavorable and favorable drug responses so we can treat patients better and we can actually design better drugs moving forward,” Dr. Kekic said.
ADVERTISEMENT
Diversity Crucial for AI
As clinical applications increase for machine learning and other forms of artificial intelligence, the diversity of data used to train these algorithms must also increase (JAMA 2020;324[12]:1212-1213; J Glob Health 2019;9[2]:010318). “The genetic data right now is predominantly white,” said Adrijana Kekic, PharmD, a pharmacogenomics clinical specialist at Mayo Clinic in Arizona. “AI systems are biased because humans are biased.”

Two projects seeking to address these concerns are the Coalition for Health AI and STANDING Together (STANdards for data Diversity, INclusivity and Generalisability) initiative, which are developing guidelines for AI healthcare technologies that would promote the use of diverse, representative data (Nat Med 2022;28[11]:2232-2233). Mayo Clinic, meanwhile, has launched a project called Platform_Validate that evaluates healthcare algorithms for efficacy, accuracy and bias in demographics such as age or ethnicity, and generates reports that echo the nutrition labels typically found on food packaging. Its aim is to “help close racial, gender and socioeconomic gaps in the delivery of care.”
Before deploying AI, it’s important to consider potential unintended legal or ethical consequences and minimize racial and other biases within the data training the algorithm, said Wei-Hsuan “Jenny” Lo-Ciganic, MSPharm, MS, PhD, a pharmacoepidemiologist at the University of Florida College of Pharmacy, in Gainesville. She stressed that AI should be considered complementary to traditional analytics for risk prediction, rather than a replacement. When an algorithm is under development, it’s important for input from end users such as pharmacists to be included early in the process to ensure the model is useful to clinical practice, she added.
—Kate Baggaley
ADVERTISEMENT
Several of her colleagues have applied ML approaches to depression. In a recent study, Dr. Kekic and her co-authors noted that “the future will almost certainly include the application of machine learning–based predictive algorithms that use both clinical and genomic information joined with other types of data” (Genet Med 2022;24[5]:1062-1072).
Considerations for Implementing AI
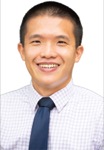
Adrian Wong, PharmD
Regarding medication safety and precision medicine, AI has “a lot of potential” but is still in the initial stages of implementation, said Adrian Wong, PharmD, MPH, FCCP, a medical ICU pharmacist at Beth Israel Deaconess Medical Center, and an adjunct associate professor of pharmacy practice at the Massachusetts College of Pharmacy and Health Sciences, both in Boston. He recently co-authored a review concerning the role of AI in pharmacy practice that examined studies from 2004 to 2022 (J Am Coll Clin Pharm 2023 Jul 10. doi.org/10.1002/jac5.1856). Among the issues under investigation were the safe use of opioids, identification of medications at risk for shortage and determining the likelihood of therapeutic warfarin dosing. Most of the studies he and his team reviewed were retrospective observational studies, although one exception concerned an ML-based early warning system for sepsis.
Among the most relevant to current clinical practice were studies that focused on determining effective therapies, Dr. Wong told Pharmacy Technology Report. “In a lot of patients, [medications] don’t work as well as they should, or they develop toxicities,” he said. A beneficial starting point for AI applications would be high-risk medications such as anticoagulants and insulin. Dr. Wong envisions an algorithm to identify patients at heightened bleeding risk with direct oral anticoagulants, or to determine the precise dose of insulin that is appropriate to manage a patient’s diabetes.
ADVERTISEMENT
Opioid Use Disorder
Machine learning (ML) algorithms may be able to predict the risk for opioid use disorder (OUD) and overdose among Medicare beneficiaries, according to pharmacoepidemiologist Wei-Hsuan “Jenny” Lo-Ciganic, MSPharm, MS, PhD. Current OUD screening approaches target many patients who are not truly at high risk. ML techniques, in contrast, could handle the numerous patient variables to generate clinically useful predictions, Dr. Lo-Ciganic and her co-investigators reported (JAMA Netw Open 2019;2[3]:e190968; PLoS One 2020;15[7]:e0235981).
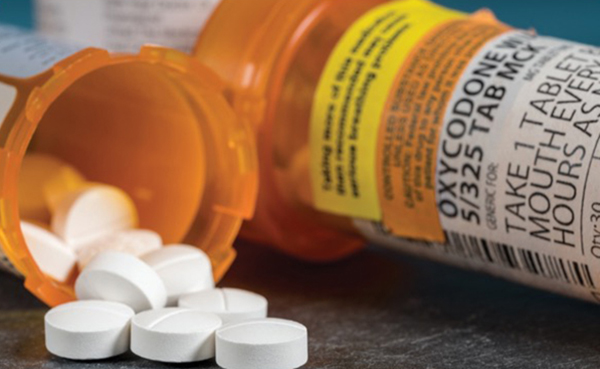
In one study, the team analyzed 560,057 fee-for-service Medicare beneficiaries with opioid prescriptions, measuring potential predictors such as health status and patterns of opioid use, and tracking opioid overdose episodes from inpatient and emergency department claims. The researchers’ algorithm classified patients into low risk (76.2%), medium risk (18.6%) and high risk (5.2%); more than 90% of overdose episodes occurred in the high- and medium-risk subgroups. “Machine-learning algorithms appear to perform well for risk prediction and stratification of opioid overdose, especially in identifying low-risk subgroups that have minimal risk of overdose,” the authors concluded.
The team extended their research to predict incident OUD among 361,527 fee-for-service Medicare beneficiaries with opioid prescriptions, based on OUD diagnosis or initiation of buprenorphine or methadone for OUD. Nearly 70% of individuals with incident OUD fell into the top two deciles of risk predicted by the algorithm, while individuals in the bottom eight deciles had minimal incident OUD (three to 28 per 10,000, vs. 36 to 301 per 10,000). The results suggest that ML algorithms improve risk prediction and stratification of incident OUD, the researchers wrote.
The studies indicate that “machine learning outperforms the traditional approach and also can help stakeholders more efficiently identify those who are at a high risk to better allocate resources,” Dr. Lo-Ciganic said.
ADVERTISEMENT
For any of these applications, the algorithm “is totally reliant on what is documented within the medical record, which is often very messy,” he said. Furthermore, it’s important to understand where the data that trained an algorithm come from and which populations are underrepresented. “AI can only be as good as the data that it’s provided to develop from,” Dr. Wong cautioned. “A lot of people who may not be familiar with artificial intelligence … might think that we can easily extrapolate what we find in these studies to clinical practice, but I’d say that it’s very hard to actually do that.”
Dr. Wong sees several roles for pharmacists in applying AI to clinical practice. Pharmacists can contribute clinical knowledge concerning medications, diagnostics and interpretation of lab results during the algorithm development phase. When implementing a model, pharmacists should ensure that it is relevant to the population they serve. Documenting side effects and adverse outcomes in the electronic health record also provides valuable data that can be used to refine the model.
It is extremely important for the pharmacy profession to become educated “on what artificial intelligence can [and] can’t do, so that we understand [its] strengths and limitations,” Dr. Wong said. “We really need to be part of the development process on these models [and] provide critical information to make sure it’s actually relevant.”
ADVERTISEMENT
Depression
Mayo Clinic researchers have used machine learning (ML) to predict how patients will respond to antidepressants. In one study, the team examined data from 264 adults with major depressive disorder who received citalopram or escitalopram. Their approach combined genomic and plasma metabolomic data with clinical measures such as symptom severity. The models predicted treatment success with over 75% accuracy (Transl Psychiatry 2021;11[1]:513).

In another study, the team examined 271 depressed children and adolescents treated with duloxetine, fluoxetine, or placebo, using ML to identify depressive symptom profile and related thresholds of improvement. The researchers found that variation in six depressive symptoms predicted treatment outcomes with greater than 70% accuracy for both drugs, and in placebo-treated patients with accuracies of 67% (J Child Psychol Psychiatry 2022;63[11]:1347-1358).
“What we can observe with that [ML] model is we basically combine clinical data with genomic data to stratify the patient in the treatment so that we can identify those who are likely to respond and give an appropriate treatment,” said pharmacogenomics clinical specialist Adrijana Kekic, PharmD.
ADVERTISEMENT
Sepsis
Sepsis is a prevalent issue for hospitalized patients, with one study of 568 adults estimating that sepsis was present in 52.8% of hospitalizations and the immediate cause of death in 34.9% (JAMA Netw Open 2019;2[2]:e187571). However, early recognition and treatment with a new machine learning (ML)-based tool can improve patient outcomes, researchers wrote in Nature Medicine (2022;28[7]:1455-1460).
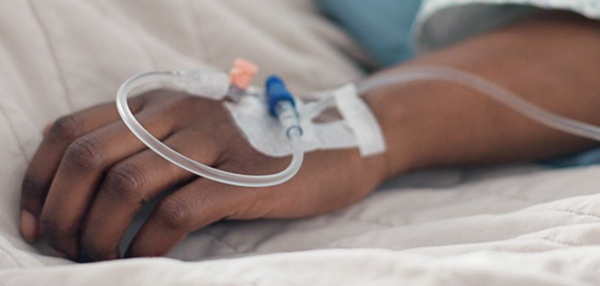
The researchers used a Targeted Real-time Early Warning System (TREWS) to monitor 590,736 patients across five hospitals in the Maryland and Washington, D.C., areas. The researchers focused on 6,877 patients with sepsis who were identified by the alert before receiving antibiotics. Patients whose alert was confirmed by a provider within three hours had reduced in-hospital mortality rates, organ failure and length of stay compared with those whose alert was not confirmed within three hours (adjusted absolute reduction: 3.3%, CI 1.7, 5.1%; adjusted relative reduction: 18.7%, CI 9.4, 27.0%). Patients who received antibiotics within three hours of triggering an alert also had a lower mortality rate than those whose treatment was delayed (adjusted risk difference: –3.54%, CI –5.52, –1.66%; adjusted relative reduction: –18.69%, CI –27.03, –9.36%; P<0.001).
“Our findings indicate that early warning systems have the potential to identify sepsis patients early and improve patient outcomes and that sepsis patients who would benefit the most from early treatment can be identified and prioritized at the time of the alert,” the researchers wrote.
In a later study, the team found that emergency department providers and providers who’d previously interacted with TREWS were more likely to engage with alerts. “Beyond efforts to improve the performance of early warning systems, efforts to improve adoption are essential to their clinical impact and should focus on understanding providers’ knowledge of, experience with and attitudes toward such systems,” the team wrote (Nat Med 2022;28[7]:1447-1454).
ADVERTISEMENT
Acute Kidney Injury
About 25% of medications used in the hospital setting can potentially cause acute kidney injury (AKI) (Crit Care Clin 2021;37[2]:303-320; bit.ly/3t4UKT0-PPN). The Multi-Hospital Electronic Decision Support for Drug-Associated AKI (MEnD-AKI) project is using ML to identify patients at risk for developing drug-associated AKI (D-AKI) (Surgery 2023;174[3]:709-714). The goal of the research is to “reduce the risk, progression and complications of D-AKI and AKI in general,” said Azra Bihorac, MD, MS, FCCM, FASN, the senior associate dean of research at the University of Florida College of Medicine and director of the UF Intelligent Critical Care Center, both in Gainesville. She told Pharmacy Technology Report that up to 60% of AKI in hospitalized patients can be related to nephrotoxins, so monitoring recent nephrotoxin exposure is an essential part of the model’s AKI predictions.

“By using a deep learning–based predictive model that can continuously be updated with new electronic health record [EHR] data and offer a real-time prediction on a patient’s AKI status for up to the next 48 hours, we can provide the physicians and pharmacists a clearer picture on which patient is at high risk for AKI and whose AKI is likely to worsen,” Dr. Bihorac said. “While we’re still testing the model’s effectiveness, the results are promising thus far.”
For each patient, the MEnD-AKI model analyzes data that include one-year EHR history, admission information, and laboratory values and other measurements taken during hospitalization. “After reviewing all these data including chronological changes in patient-specific measures of interest, a prediction is made of the patient’s AKI stage,” Dr. Bihorac said.
The research is intended to assist pharmacists in their efforts to reduce or mitigate D-AKI, she emphasized. “It would be unrealistic to expect a clinician or pharmacist to assess this vast amount of data in such a short time frame, which is why AI can be very helpful,” Dr. Bihorac said. “The ability of AI to provide predictions based on so much EHR data could be a great asset to pharmacists’ ability to address D-AKI and adjust drug dosing of other forms of AKI to improve patient care.”
Dr. Bihorac received research funding from Astute Medical and the National Institutes of Health. She also reported Method and Apparatus for Pervasive Patient Monitoring, U.S. Patent No. 11424028B2, date of patent Aug. 23, 2022; Systems and Methods for Providing an Acuity Score for Critically Ill or Injured Patients, U.S. Patent Application Publication 20220044809A1, publication date Feb. 10, 2022; and Method and Apparatus for Prediction of Complications after Surgery, U.S. Patent Application Publication No. 20200161000A1, publication date May 21, 2020. Drs. Kekic and Wong reported no relevant financial disclosures. Dr. Lo-Ciganic is supported by the National Institute on Drug Abuse National Institute of Mental Health, and Richard King Mellon Foundation. She has also received research funding from Bristol Myers Squibb and Merck.